- The AI Odyssey
- Posts
- Explainable AI: Unlocking the Black Box
Explainable AI: Unlocking the Black Box
How Transparency is Key to AI Acceptance

Imagine yourself driving down the highway at 70 miles per hour in a self-driving vehicle. The vehicle suddenly swerves to avoid a hazard in the road. But why did it decide that way? What aspects did the AI algorithm consider? And how do you know that you made the right choice?
The need for transparency and explainability in machine learning is one of the major issues that the development and application of artificial intelligence (AI) in the modern world must deal with. It’s critical that we comprehend how these algorithms function and how they make decisions as AI becomes more prevalent across a variety of industries and applications. This article will examine the rise of explainable AI and the significance of transparency in the machine learning industry.
Let’s start by defining what “explainable AI” means. Explainable AI basically means that AI algorithms can give precise, understandable justifications for the decisions they make. This is crucial because, as AI develops, it can become challenging to comprehend how it arrives at its conclusions. For instance, deep learning algorithms may have hundreds or even thousands of interconnected layers, which makes it challenging for humans to comprehend the algorithm’s operation.

Particularly in industries with high stakes and unpredictable outcomes, such as healthcare or finance, this lack of transparency and explainability can be a serious issue. Consider a medical AI system that suggests a patient’s course of treatment. It can be challenging for doctors and patients to understand why a specific treatment was advised and whether it is the best course of action if the algorithm is opaque and difficult to understand.
Another illustration comes from the financial sector, where AI is increasingly being used for tasks like risk management and fraud detection. It’s critical to have a clear justification for any decision made by an AI algorithm that flags a specific transaction as possibly fraudulent. Otherwise, it might be challenging to determine whether the algorithm is operating as intended or if it has faults.
So why is explainable AI becoming more prevalent? There are several of them. First and foremost, there is an increasing need for accountability and transparency as AI becomes more complex and popular. It can be challenging to know whether AI algorithms are performing as intended without precise explanations of how they operate.

This problem also has an ethical component. It’s critical that we comprehend how these systems make decisions and what considerations they make as AI becomes more integrated into our daily lives. This can assist in preventing discrimination and bias and guarantee that AI is being used fairly and responsibly.
Another practical point is that explainable AI may actually improve the performance of AI algorithms. It may be simpler to find and correct biases or errors in the algorithm if decision-making processes are clearly explained. It may also make it simpler to optimize the algorithm’s performance.
So how can we make machine learning more transparent and understandable? Several approaches are being researched right now. Utilizing “interpretable” machine learning models, which are intended to be more transparent and understandable, is one strategy. These models are frequently based on decision trees or other rule-based approaches that are simple to understand and are typically simpler than more complicated deep learning algorithms.
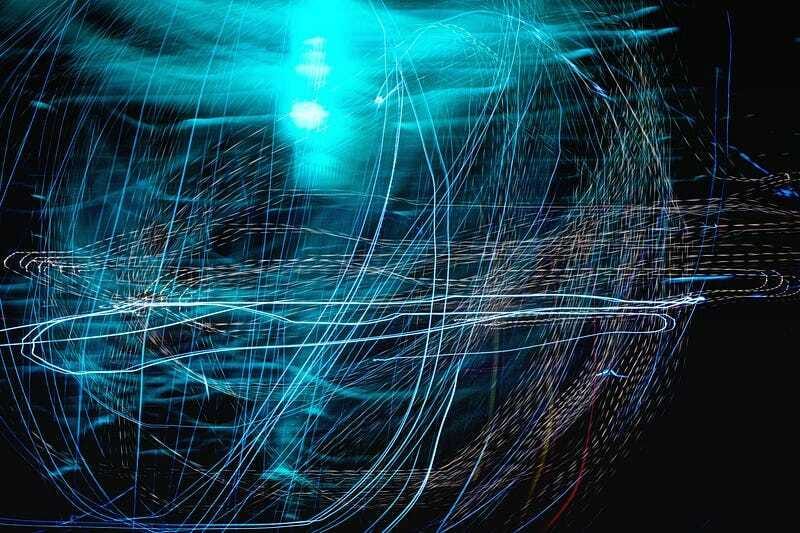
Utilizing strategies like “counterfactual explanation,” which creates fictitious scenarios to help explain how a decision was made, is another strategy. An AI system in medicine, for instance, might create a fictitious patient who is comparable to the real patient but received a different treatment recommendation. It can be easier to understand how the algorithm arrived at its recommendation by looking at how it might have decided differently in that situation.
In summary, the development of explainable AI is crucial for machine learning. It’s critical that we comprehend how these algorithms function and how they make decisions as AI spreads and becomes more sophisticated. We can guarantee that AI is being used fairly and responsibly while also maximizing its effectiveness and potential by placing a high priority on transparency and accountability. Therefore, you can feel comfortable knowing why a self-driving car is making a particular choice the next time you’re in one.
Enjoyed the read? Join our (free) thriving community of 50,000+ readers, including professionals from top companies like Apple, Amazon, Google, Meta, Microsoft, and more.